Enhancing CLIP For Semantic Segmentation: Minimal Modifications + CSA
Does the future of visual understanding lie in the subtle art of modification, or the revolutionary power of reinvention? Recent advancements in the field of semantic segmentation suggest a compelling answer, hinting at a paradigm shift within the realm of computer vision, where the potential for groundbreaking innovation is realized by building upon pre-existing foundations.
In a world increasingly saturated with digital content, the ability of machines to "see" and interpret images with human-like accuracy has become paramount. Semantic segmentation, the process of assigning a label to every pixel in an image, is a cornerstone of this capability. It allows computers to not only identify objects but also to understand their boundaries and relationships, paving the way for a new era of applications, from self-driving cars to medical diagnostics. But how can we achieve this level of understanding, while minimizing the computational cost and the need for vast datasets?
The quest to unlock the full potential of visual understanding has led researchers to explore innovative approaches. One such approach involves leveraging the power of pre-trained models. These models, trained on massive datasets, have already learned a wealth of visual information. The challenge lies in adapting these pre-trained models to the specific task of semantic segmentation. By making minimal modifications, researchers aim to tap into the existing knowledge base, reducing the need for extensive training and accelerating the development process. The goal is to enhance existing models without the need for complex adjustments.
One exciting avenue of research is the use of "CLIP" models. CLIP (Contrastive Language-Image Pre-training) models have demonstrated remarkable ability in understanding the relationship between images and text. Their ability to connect visual concepts with textual descriptions makes them ideal candidates for semantic segmentation. The core concept of CLIP is to learn visual representations that are closely aligned with textual descriptions. This means that the model can identify objects and scenes in images based on their textual labels. Researchers are now investigating how to leverage the power of CLIP models for semantic segmentation, with a focus on minimizing modifications to the pre-trained models.
A significant advancement has come with the innovative application of a "Contextual Self-Attention" (CSA) mechanism. By re-thinking the concept of self-attention, a core component of many deep learning models, researchers discovered that CLIP could be adapted to handle complex tasks. The CSA mechanism provides a training-free adaptation method for zero-shot semantic segmentation. The approach involves replacing the traditional self-attention block in the CLIP visual encoder with a novel CSA module. Then, it reuses the pre-trained query, key, and value projection matrices, providing an adaptive method for CLIP's zero-shot semantic segmentation. This is a powerful way to leverage the knowledge already present in the pre-trained models.
The results of this approach are remarkable. The CSA mechanism has demonstrated significant improvements in semantic segmentation performance. Experiments on eight semantic segmentation benchmarks show an impressive average zero-shot mIoU of 38.2%, considerably higher than the existing SOTA of 33.9% and the 14.1% achieved by standard CLIP. This highlights the potential of this approach to unlock the power of semantic segmentation, using methods that demand fewer adjustments to the models.
This research opens new opportunities to apply this technology. One such example is "Clip TV," a multi-platform internet television service offering over 100 television channels and over 3,000 hours of licensed Hollywood films. Clip TV offers a 30-day free trial, allowing users to experience content without any limits. The platform aims to provide a wide range of entertainment options to its users.
Further research efforts are being made to enhance the system and refine the approach even more. By minimizing changes to the pre-trained models, researchers are demonstrating that significant gains can be made in semantic segmentation. This research presents a promising direction for the future of semantic segmentation, paving the way for more efficient, accurate, and adaptable visual understanding models.
The challenges in this field are clear, as the performance degrade when applying a consistent evaluating code with other methods (tcl, groupvit.). However, the innovative approaches are driving the field forward.
In other news, the world of entertainment is constantly evolving, and new narratives emerge. The show "Slip" follows Mae as she navigates a surreal journey through parallel universes. In a marriage that is failing, Mae must go on an unusual journey to different realities. In these realities, Mae is married to different people, and she's trying to find her way back to her original partner and, ultimately, find herself.
It is a series that received positive reviews and nominations. It premiered and was removed from the Roku Channel in April 2023. Mae meets Rose, who gives her information that she may not be ready to handle, and attempts to bring Gina into her nightmare. In another universe, Mae must deal with unfamiliar circumstances while looking for answers from Monk Dawa. Mae and Elijah have been together for 13 years, and there is love, but no fire.
For those interested in staying up to date with the latest in AI and computer vision, following key sources of information is essential. The @cver official Zhihu account is an invaluable resource for accessing high-quality, cutting-edge work.
One can find more details for Clip TV, the multi-platform internet television service. It provides more than 100 TV channels, over 3,000 hours of 100% licensed Hollywood movies, a 30-day free trial for unlimited access, providing a comprehensive entertainment experience.
Additionally, the platform "clictv.es" offers the latest news, premieres, and features. Clip TV also offers a range of entertainment content, including television, movies, comedy videos, sports, and TV shows, all of which are 100% licensed.
Clip TV has a mission to deliver compelling entertainment content from television, movies, and other media. It aims to provide a wide range of content, including over 3,000 hours of Hollywood movies and television shows. The platform's goal is to make entertainment easily accessible to users.
Further research on the topic focuses on the use of "CSA" (Contextual Self-Attention) and how it can enhance the capabilities of CLIP models. These studies explore techniques to fine-tune existing models, which is crucial for various applications, including semantic segmentation, image classification, and object detection.
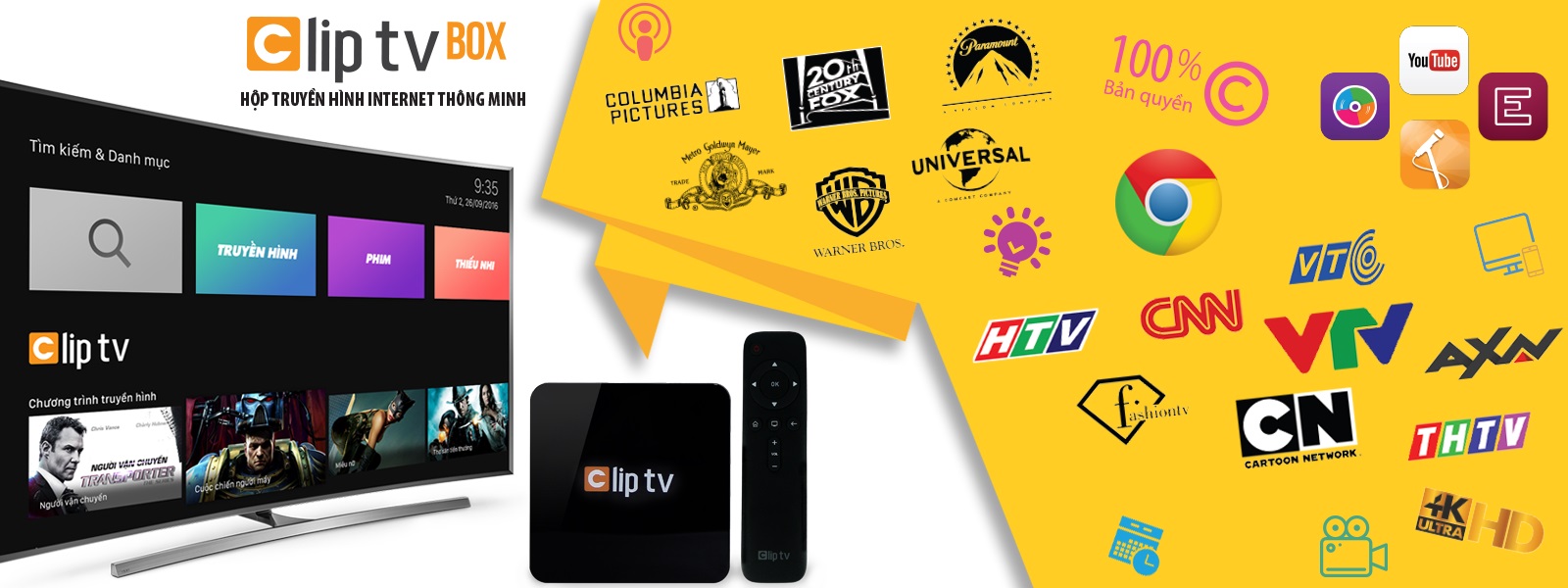
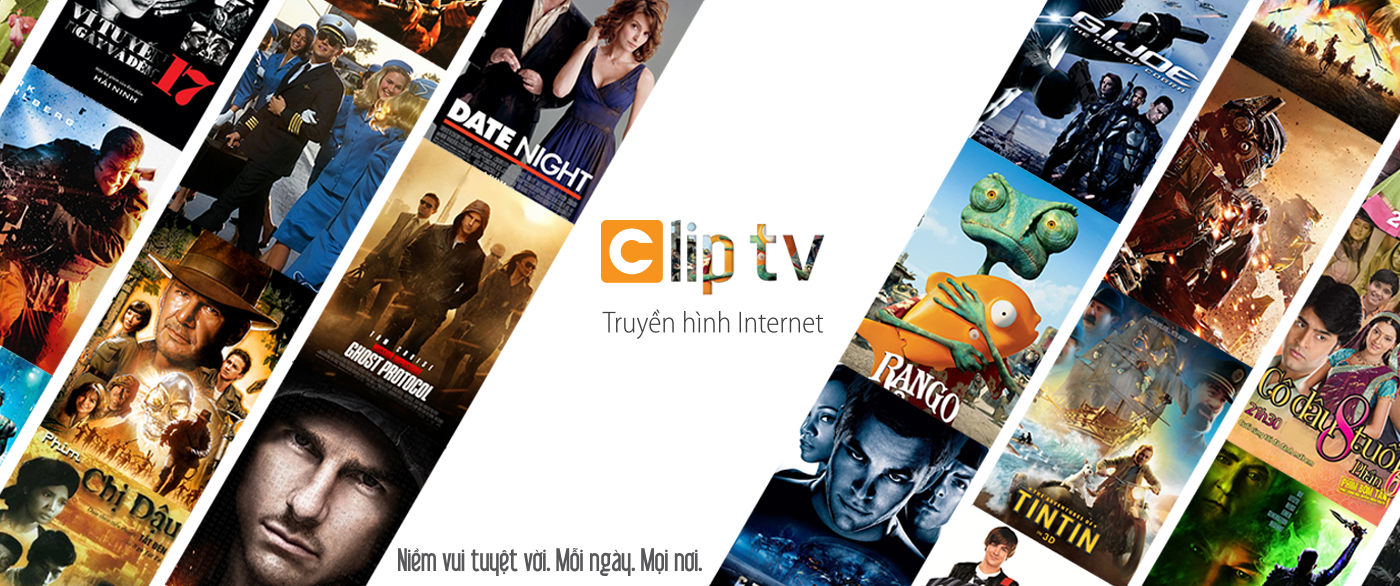
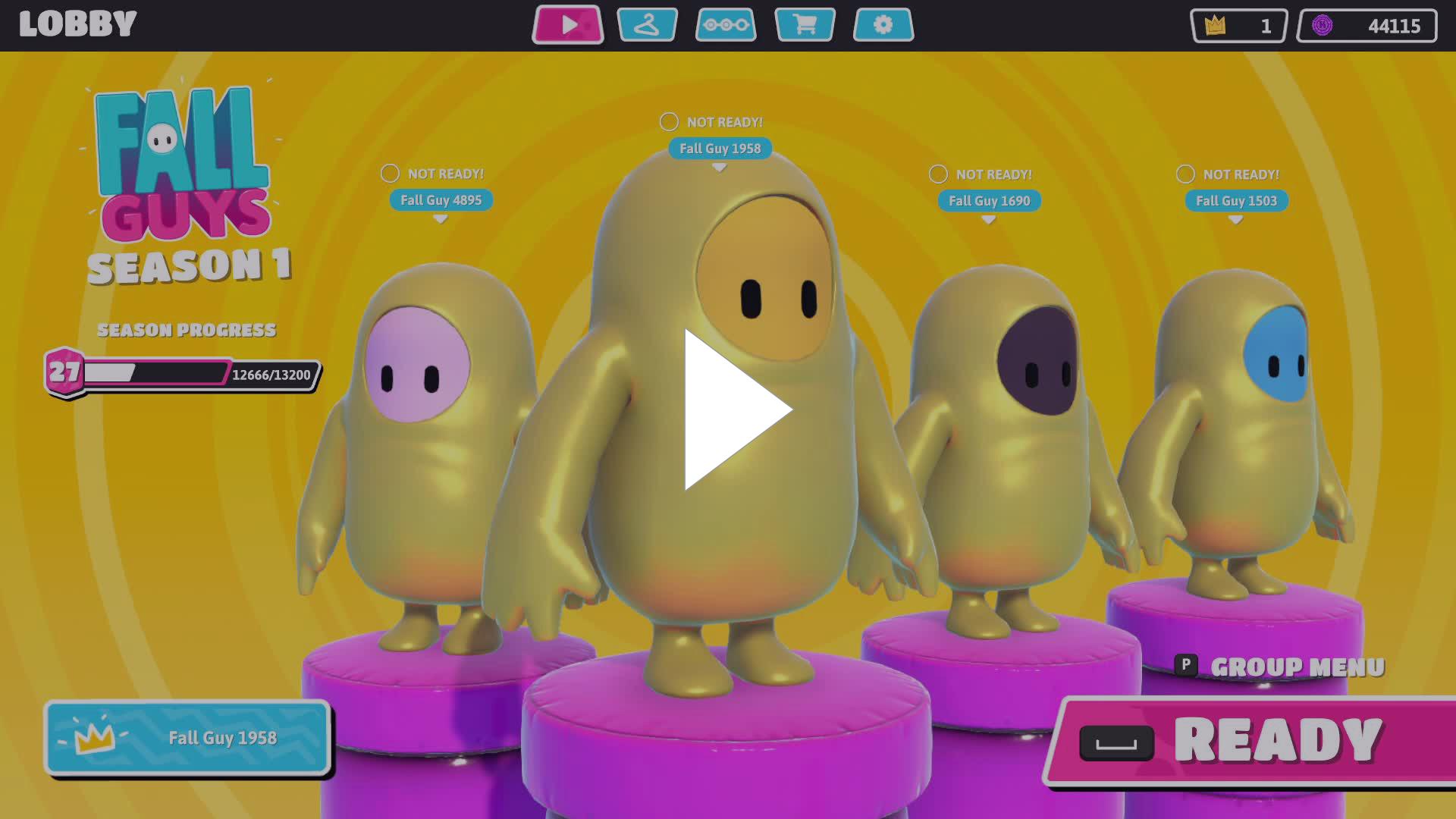